![Tek Styz PRO USB-C HDMI Works for Samsung Galaxy Tab A7 10.4/A 8.4 (2020)/10.1 (2019) at 4k with Power Port, 6ft Cable at Full 2160p@60Hz, 6Ft/2M Cable [Thunderbolt 3 Compatible] - Walmart.com Tek Styz PRO USB-C HDMI Works for Samsung Galaxy Tab A7 10.4/A 8.4 (2020)/10.1 (2019) at 4k with Power Port, 6ft Cable at Full 2160p@60Hz, 6Ft/2M Cable [Thunderbolt 3 Compatible] - Walmart.com](https://i5.walmartimages.com/asr/be5f77e2-607d-43e9-8f71-dbaaa1386cfc.ab22966d05f478e57e5b99e9be802840.jpeg)
Tek Styz PRO USB-C HDMI Works for Samsung Galaxy Tab A7 10.4/A 8.4 (2020)/10.1 (2019) at 4k with Power Port, 6ft Cable at Full 2160p@60Hz, 6Ft/2M Cable [Thunderbolt 3 Compatible] - Walmart.com
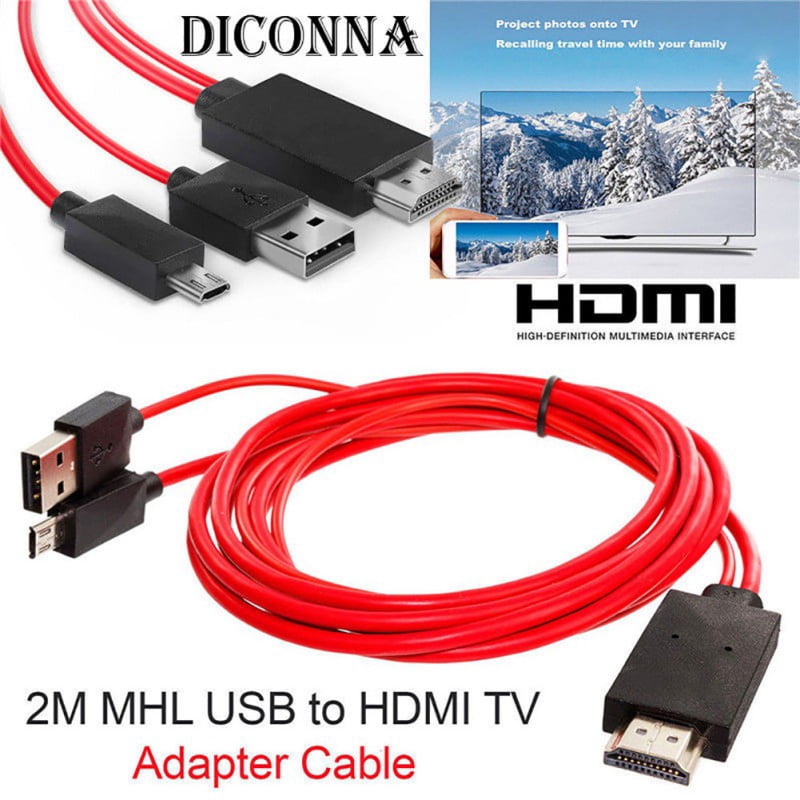
7 Feet MHL Micro USB for HDMI Adapter Converter Cable 1080P HDTV Only Compatible with Samsung Galaxy S3 S4 S5 Note 3/Samsung Galaxy Tab 3 8.0 T310, Tab 3 10.1 P5210 - Walmart.com
![USB-C/PD 4k HDMI Cable Compatible with Samsung Galaxy Tab S5e/Galaxy Tab S6 with Full 2160p@30Hz, 6Ft/2M Cable [Gray, Thunderbolt 3 Compatible] - Walmart.com USB-C/PD 4k HDMI Cable Compatible with Samsung Galaxy Tab S5e/Galaxy Tab S6 with Full 2160p@30Hz, 6Ft/2M Cable [Gray, Thunderbolt 3 Compatible] - Walmart.com](https://i5.walmartimages.com/asr/43a7b586-35cd-4876-a340-16804c527a1f.460a9029c4512d53a6726939c8dd0139.jpeg)
USB-C/PD 4k HDMI Cable Compatible with Samsung Galaxy Tab S5e/Galaxy Tab S6 with Full 2160p@30Hz, 6Ft/2M Cable [Gray, Thunderbolt 3 Compatible] - Walmart.com
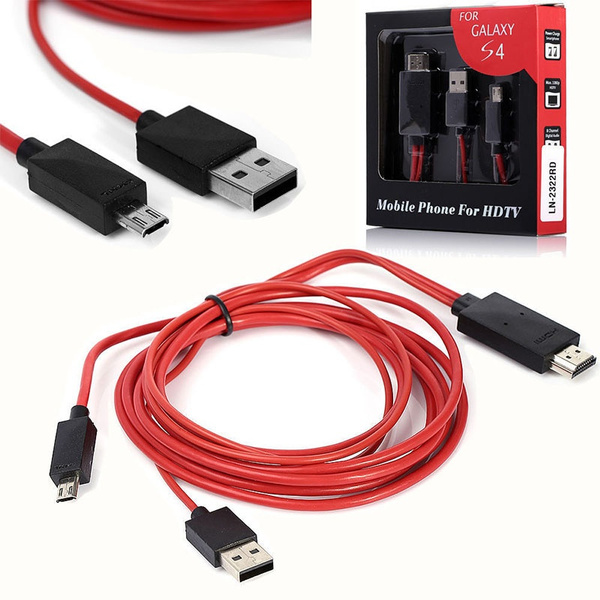
MHL Micro USB to HDMI Adapter Cable 2m 11pin 1080P HDTV for Samsung Galaxy S5 S4 S3 Note 3 2 Galaxy Tab 3 Tab Pro T310 | Wish

Mhl Usb Hdmi Tv Cable Adapter For Samsung Galaxy Tab 3 10.1 Gt P5200 P5210 P5220(mhl-11p-2m-040) - Mobile Phone Cables - AliExpress

Amazon.com: Cybertech MHL Micro USB to HDMI HDTV Replacement Adapter Compatible for Samsung Galaxy Tab 3 8.0 10.1-2 Meters Extra Long Cable (Charge Your Phone While Watching HD Video) : Cell Phones & Accessories

Amazon.com: MHL to HDMI Cable Adapter AV TV Video Cord for Samsung Galaxy Tab 3 8.0'' 10.1" Inch GT-P5210 Tablet : Electronics

Connect a Samsung tablet to a monitor via a HDMI cable and the optional HDMI (HDTV) adapters - YouTube

Amazon.com: MHL to HDMI Cable Adapter AV TV Video Cord for Samsung Galaxy Tab 3 8.0'' 10.1" Inch GT-P5210 Tablet : Electronics
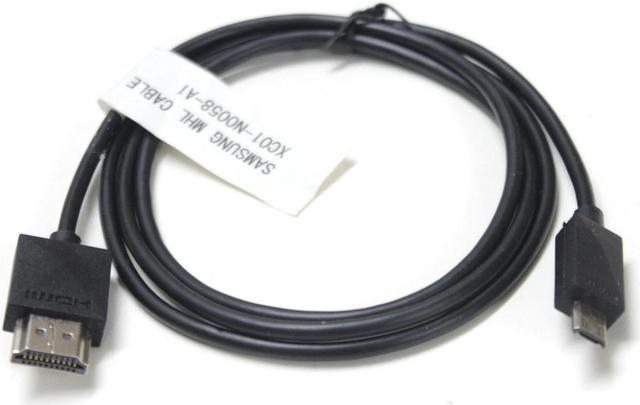
OIAGLH MHL 11-pin Micro USB to HDMI Cable for Galaxy S3 S4 S5, Note 2 3 4, Galaxy Tab 3, Tab S, Tab Pro USB Cables - Newegg.com
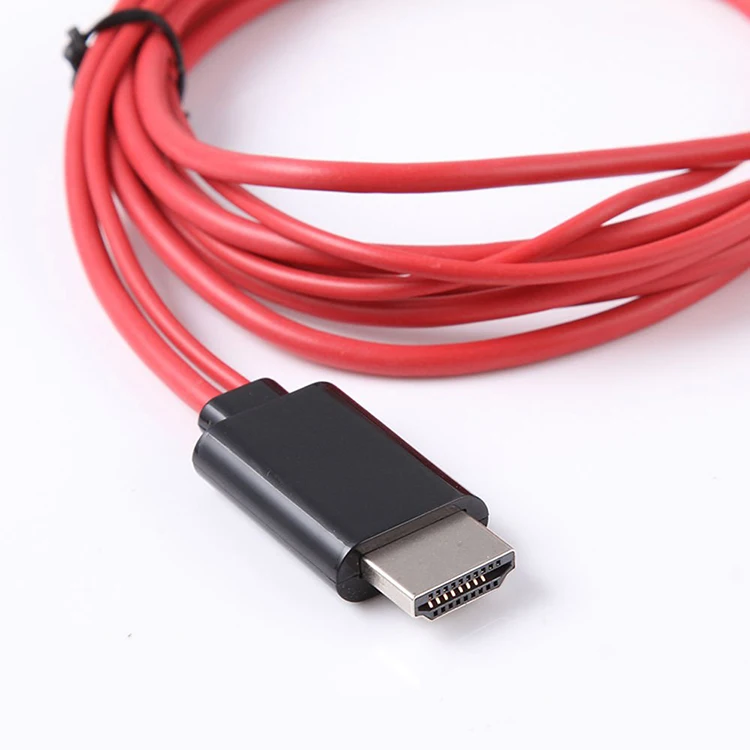
Wholesale Micro USB to HDMI Cable Cord 1080P HDTV Adapter for Samsung Galaxy S5, S4, S3, Note 3, Note 2, Galaxy Tab 3 8.0, Tab 3 10.1 From m.alibaba.com
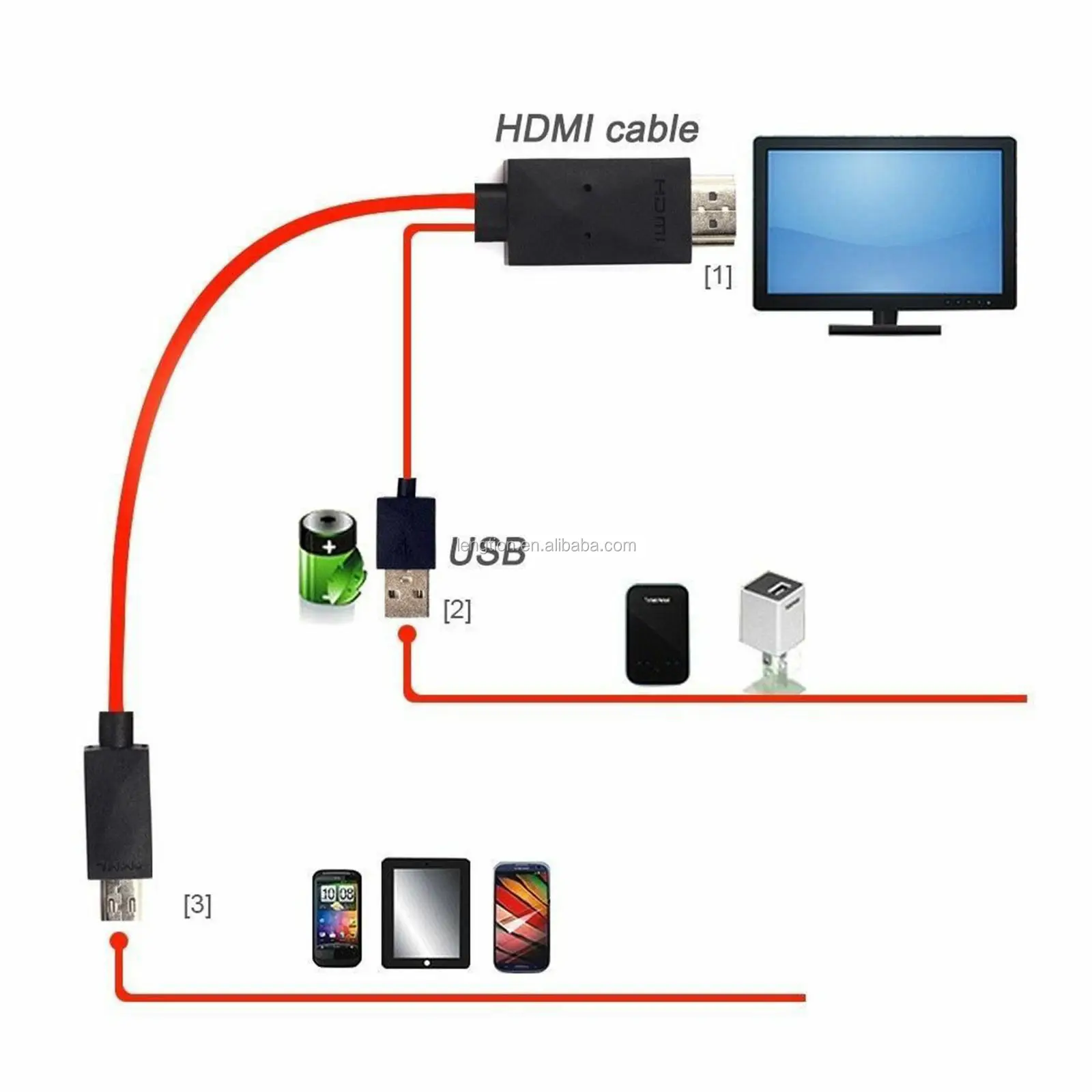